WMT/AW integration To get the most out of your site data, you should connect all of your Google Suite products. In this chapter, we'll walk through how to easily link AdWords and Search Console to Analytics.
- Wmt Aw Integration Group
- Wmt Aw Integration Solutions
- Wmt Aw Integration Services
- Wmt Aw Integration Inc
Amber Waves Integration offers quality integration and automation services for the Agricultural Processing, Manufacturing, and Food & Beverage industries. We have the ability to take off the shelf hardware and customize it for your needs. CNBC is the world leader in business news and real-time financial market coverage. Find fast, actionable information.
Home
This conference builds on a series of annual workshops and conferences on statistical machine translation, going back to 2006:
- the NAACL-2006 Workshop on Statistical Machine Translation,
- the ACL-2007 Workshop on Statistical Machine Translation,
- the ACL-2008 Workshop on Statistical Machine Translation,
- the EACL-2009 Workshop on Statistical Machine Translation,
- the ACL-2010 Workshop on Statistical Machine Translation
- the EMNLP-2011 Workshop on Statistical Machine Translation,
- the NAACL-2012 Workshop on Statistical Machine Translation,
- the ACL-2013 Workshop on Statistical Machine Translation,
- the ACL-2014 Workshop on Statistical Machine Translation,
- the EMNLP-2015 Workshop on Statistical Machine Translation,
- the First Conference on Machine Translation (at ACL-2016),
- the Second Conference on Machine Translation (at EMNLP-2017),
- the Third Conference on Machine Translation (at EMNLP-2018).
IMPORTANT DATES
Release of training data for shared tasks | January/February, 2019 |
Evaluation periods for shared tasks | April, 2019 |
Paper submission deadline | May 17, 2019 |
Paper notification | June 7, 2019 |
Camera-ready version due | June 17, 2019 |
Conference in Florence | August 1-2, 2019 |
OVERVIEW
This year's conference will feature the following shared tasks:
- a news translation task
- a biomedical translation task ,
- a similar language translation task,
- an automatic post-editing task,
- a metrics task (assess MT quality given reference translation),
- a quality estimation task (assess MT quality without access to any reference),
- a robust translation task, and
- a parallel corpus filtering task.
In addition to the shared tasks, the conference will also feature scientific papers on topics related to MT. Topics of interest include, but are not limited to:
- MT models (neural, statistical etc. )
- analysis of neural models for MT
- using comparable corpora for MT
- selection and preparation of training data for MT
- incorporating linguistic information into MT
- decoding
- system combination
- error analysis
- manual and automatic methods for evaluating MT
- quality estimation for MT
REGISTRATION AND VISA INFORMATION
These will both be handled by ACL 2019.NEWS TRANSLATION TASK
This shared task will examine translation between the following language pairs:
- English-Chinese and Chinese-English
- English-Czech
- English-Finnish and Finnish-English
- English-German and German-English
- English-Gujarati and Gujarati-English
- English-Kazakh and Kazakh-English
- English-Lithuanian and Lithuanian-English
- English-Russian and Russian-English
- French-German and German-French
- Language Pairs
- This year we introduce two low-resource language pairs (English to/from Kazakh and Gujarati) plus a further Baltic language pair (English to/from Lithuanian) and a non-English pair (French to/from German).
- Document level MT
- We encourage the use of document-level models for English to German and for Chinese to English. We will ensure that the data for de-en has document boundaries in it. We will evaluate both these pairs with the context visible to evaluators.
- Data sets
- We will release parallel and monolingual data for all languages, updated where possible. For the low-resource language pairs, we encourage participants to explore additional data sets (sharing these with the community whenever possible).
BIOMEDICAL TRANSLATION TASK
In this fourth edition of this task, we will evaluate systems for the translation of biomedical documents for the following languages pairs:
- English-French and French-English
- English-Portuguese and Portuguese-English
- English-Spanish and Spanish-English
- English-German and German-English
- English-Chinese and Chinese-English
Parallel corpora will be available for all language pairs but also monoligual corpora for some languages. Evaluation will be carried out both automatically and manually.
ROBUSTNESS TRANSLATION TASK
This year we have a new task focusing on robustness of machine translation to noisy input text. We will evaluate translation of the following language pairs:
- English-French and French-English
- English-Japanese and Japanese-English
We release both parallel and monolingual data for all languages pairs. You can find more details in the task page.
SIMILAR LANGUAGE TRANSLATION TASK
This shared task will focus on the translation between three pairs of similar languages:
- Czech - Polish (Slavic languages)
- Hindi - Nepali (Indo-Aryan languages)
- Spanish - Portuguese (Romance languages)
AUTOMATIC POST-EDITING TASK
This shared task will examine automatic methods for correcting errors produced by machine translation (MT) systems. Automatic Post-editing (APE) aims at improving MT output in black box scenarios, in which the MT system is used 'as is' and cannot be modified.From the application point of view APE components would make it possible to:
- Cope with systematic errors of an MT system whose decoding process is not accessible
- Provide professional translators with improved MT output quality to reduce (human) post-editing effort
In this fifth edition of the task, the evaluation will focus on two subtasks:
- English-German (IT domain), with MT segments produced by a neural system
- English-Russian (IT domain), with MT segments produced by a neural system
METRICS TASK
See task page.QUALITY ESTIMATION TASK
Quality estimation systems aim at producing an estimate on the quality of a given translation at system run-time, without access to a reference translation. This topic is particularly relevant from a user perspective. Among other applications, it can:help decide whether a given translation is good enough for publishing as is,filter out sentences that are not good enough for post-editing,select the best translation among options from multiple MT and/or translation memory systems,inform readers of the target language of whether or not they can rely on a translation,andspot parts (words or phrases) of a translation that are potentially incorrect.
This year's WMT shared task on quality estimation consists of three tracks according to the specific needs QE satisfies:
Task 1: estimating post-editing effort on word and sentence level,
Task 2: performing MT output diagnostics on document and word/phrase level and
Task 3: scoring MT outputs just like metrics do, but without a reference.
We provide new train and test sets based on neural machine translation from English to Russian, German and French.We also supply the participants with baseline systems and an automatic evaluation environment for submitting the results.
See the task pagefor further details.
PAPER SUBMISSION INFORMATION
Submissions will consist of regular full papers of 6-10 pages, plus additional pages for references, formatted following the ACL 2019 guidelines. Supplementary material can be added to research papers. In addition, shared task participants will be invited to submit short papers (suggested length: 4-6 pages, plus references) describing their systems or their evaluation metrics. Both submission and review processes will be handled electronically. Note that regular papers must be anonymized, while system descriptions should not be.
Research papers that have been or will be submitted to other meetings or publications must indicate this at submission time, and must be withdrawn from the other venues if accepted and published at WMT 2019. We will not accept for publication papers that overlap significantly in content or results with papers that have been or will be published elsewhere. It is acceptable to submit work that has been made available as a technical report (or similar, e.g. in arXiv) without citing it. This double submission policy only applies to research papers, so system papers can have significant overlap with other published work, if it is relevant to the system description.
We encourage individuals who are submitting research papers to evaluate their approaches using the training resources provided by this conference and past workshops, so that their experiments can be repeated by others using these publicly available corpora.
POSTER FORMAT
Poster size:Posters should be no wider than 91 cm (36 in) and no higher than 122 cm (48 in), in vertical/portrait orientation. A0 paper (in vertical/portrait orientation) meets these requirements.
Poster printing option: If you cannot print your poster before travelling, there will be an A3 printer at the conference registration desk which can be used. If you need to use this option, please contact the information desk early to make sure there is time to print the posters before your session. Larger sizes (e.g. A0) can be printed in photo shops in Florence (note that shops will be closed on Sunday). The registration desk will provide information about the shops closest to the conference venue.
ANNOUNCEMENTS
Subscribe to to the announcement list for WMT by entering your e-mail address below. This list will be used to announce when the test sets are released, to indicate any corrections to the training sets, and to amend the deadlines as needed. |
You can read past announcements on the Google Groups page for WMT. These alsoinclude an archive of announcements from earlier workshops. |
INVITED TALK
Marine Carpuat (University of Maryland): Semantic, Style & Other Data Divergences in Neural Machine Translation (slides)ORGANIZERS
Ondřej Bojar (Charles University in Prague)Rajen Chatterjee (Apple)
Christian Federmann (MSR)
Mark Fishel (University of Tartu)
Yvette Graham (DCU)
Barry Haddow (University of Edinburgh)
Matthias Huck (LMU Munich)
Antonio Jimeno Yepes (IBM Research Australia)
Philipp Koehn (University of Edinburgh / Johns Hopkins University)
André Martins (Unbabel)
Christof Monz (University of Amsterdam)

Matteo Negri (FBK)

Wmt Aw Integration Group
Mariana Neves (German Federal Institute for Risk Assessment)Matt Post (Johns Hopkins University)
Marco Turchi (FBK)
Karin Verspoor (University of Melbourne)
PROGRAM COMMITTEE
- Tamer Alkhouli (RWTH Aachen University)
- Antonios Anastasopoulos (Carnegie Mellon University)
- Yuki Arase (Osaka University)
- Mihael Arcan (INSIGHT, NUI Galway)
- Duygu Ataman (Fondazione Bruno Kessler - University of Edinburgh)
- Eleftherios Avramidis (German Research Center for Artificial Intelligence (DFKI))
- Amittai Axelrod (Didi Chuxing)
- Parnia Bahar (RWTH Aachen University)
- Ankur Bapna (Google AI)
- Petra Barancikova (Charles University in Prague, Faculty of Mathematics and Physics)
- Joost Bastings (University of Amsterdam)
- Rachel Bawden (University of Edinburgh)
- Meriem Beloucif (University of Hamburg)
- Graeme Blackwood (IBM Research AI)
- Frédéric Blain (University of Sheffield)
- Chris Brockett (Microsoft Research)
- Bill Byrne (University of Cambridge)
- Elena Cabrio (Université Côte d’Azur, Inria, CNRS, I3S, France)
- Marine Carpuat (University of Maryland)
- Francisco Casacuberta (Universitat Politècnica de València)
- Sheila Castilho (Dublin City University)
- Rajen Chatterjee (Apple Inc)
- Boxing Chen (Alibaba)
- Colin Cherry (Google)
- Mara Chinea-Rios (Universitat Politècnica de València)
- Chenhui Chu (Osaka University)
- Ann Clifton (Spotify)
- Marta R. Costa-jussà (Universitat Politècnica de Catalunya)
- Josep Crego (SYSTRAN)
- Raj Dabre (NICT)
- Steve DeNeefe (SDL Research)
- Michael Denkowski (Amazon)
- Mattia A. Di Gangi (Fondazione Bruno Kessler)
- Miguel Domingo (Universitat Politècnica de València)
- Kevin Duh (Johns Hopkins University)
- Marc Dymetman (Naver Labs Europe)
- Hiroshi Echizen'ya (Hokkai-Gakuen University)
- Sergey Edunov (Faceook AI Research)
- Marcello Federico (Amazon AI)
- Yang Feng (Institute of Computing Technology, Chinese Academy of Sciences)
- Andrew Finch (Apple Inc.)
- Orhan Firat (Google AI)
- George Foster (Google)
- Alexander Fraser (Ludwig-Maximilians-Universität München)
- Atsushi Fujita (National Institute of Information and Communications Technology)
- Juri Ganitkevitch (Google)
- Mercedes García-Martínez (Pangeanic)
- Ekaterina Garmash (KLM Royal Dutch Airlines)
- Jesús González-Rubio (WebInterpret)
- Isao Goto (NHK)
- Miguel Graça (RWTH Aachen University)
- Roman Grundkiewicz (School of Informatics, University of Edinburgh)
- Mandy Guo (Google)
- Jeremy Gwinnup (Air Force Research Laboratory)
- Thanh-Le Ha (Karlsruhe Institute of Technology)
- Nizar Habash (New York University Abu Dhabi)
- Gholamreza Haffari (Monash University)
- Greg Hanneman (Amazon)
- Christian Hardmeier (Uppsala universitet)
- Eva Hasler (SDL Research)
- Yifan He (Alibaba Group)
- John Henderson (MITRE)
- Christian Herold (RWTH Aachen University)
- Felix Hieber (Amazon Research)
- Hieu Hoang (University of Edinburgh)
- Vu Cong Duy Hoang (The University of Melbourne)
- Bojie Hu (Tencent Research, Beijing, China)
- Junjie Hu (Carnegie Mellon University)
- Mika Hämäläinen (University of Helsinki)
- Gonzalo Iglesias (SDL)
- Kenji Imamura (National Institute of Information and Communications Technology)
- Aizhan Imankulova (Tokyo Metropolitan University)
- Julia Ive (University of Sheffield)
- Marcin Junczys-Dowmunt (Microsoft)
- Shahram Khadivi (eBay)
- Huda Khayrallah (Johns Hopkins University)
- Douwe Kiela (Facebook)
- Yunsu Kim (RWTH Aachen University)
- Rebecca Knowles (Johns Hopkins University)
- Julia Kreutzer (Department of Computational Linguistics, Heidelberg University)
- Shankar Kumar (Google)
- Anoop Kunchukuttan (Microsoft AI and Research)
- Surafel Melaku Lakew (University of Trento and Fondazione Bruno Kessler)
- Ekaterina Lapshinova-Koltunski (Universität des Saarlandes)
- Alon Lavie (Amazon/Carnegie Mellon University)
- Gregor Leusch (eBay)
- William Lewis (Microsoft Research)
- Jindřich Libovický (Charles University)
- Patrick Littell (National Research Council of Canada)
- Qun Liu (Huawei Noah's Ark Lab)
- Samuel Läubli (University of Zurich)
- Pranava Madhyastha (Imperial College London)
- Andreas Maletti (Universität Leipzig)
- Saab Mansour (Apple)
- Sameen Maruf (Monash University)
- Arne Mauser (Google, Inc)
- Arya D. McCarthy (Johns Hopkins University)
- Antonio Valerio Miceli Barone (The University of Edinburgh)
- Paul Michel (Carnegie Mellon University)
- Aaron Mueller (The Johns Hopkins University)
- Kenton Murray (University of Notre Dame)
- Tomáš Musil (Charles University)
- Mathias Müller (University of Zurich)
- Masaaki Nagata (NTT Corporation)
- Toshiaki Nakazawa (The University of Tokyo)
- Preslav Nakov (Qatar Computing Research Institute, HBKU)
- Graham Neubig (Carnegie Mellon University)
- Jan Niehues (Maastricht University)
- Nikola Nikolov (University of Zurich and ETH Zurich)
- Xing Niu (University of Maryland)
- Tsuyoshi Okita (Kyushuu institute of technology)
- Daniel Ortiz-Martínez (Technical University of Valencia)
- Myle Ott (Facebook AI Research)
- Santanu Pal (Saarland University)
- Carla Parra Escartín (Unbabel)
- Pavel Pecina (Charles University)
- Stephan Peitz (Apple)
- Sergio Penkale (Lingo24)
- Mārcis Pinnis (Tilde)
- Martin Popel (Charles University, Faculty of Mathematics and Physics, UFAL)
- Maja Popović (ADAPT Centre @ DCU)
- Matīss Rikters (Tilde)
- Annette Rios (Institute of Computational Linguistics, University of Zurich)
- Jan Rosendahl (RWTH Aachen University)
- Raphael Rubino (DFKI)
- Devendra Sachan (CMU / Petuum Inc.)
- Elizabeth Salesky (Carnegie Mellon University)
- Hassan Sawaf (Amazon Web Services)
- Jean Senellart (SYSTRAN)
- Rico Sennrich (University of Edinburgh)
- Patrick Simianer (Lilt)
- Linfeng Song (University of Rochester)
- Felix Stahlberg (University of Cambridge, Department of Engineering)
- Dario Stojanovski (LMU Munich)
- Katsuhito Sudoh (Nara Institute of Science and Technology (NAIST))
- Felipe Sánchez-Martínez (Universitat d'Alacant)
- Aleš Tamchyna (Charles University in Prague, UFAL MFF)
- Gongbo Tang (Uppsala University)
- Jörg Tiedemann (University of Helsinki)
- Antonio Toral (University of Groningen)
- Ke Tran (Amazon)
- Marco Turchi (Fondazione Bruno Kessler)
- Ferhan Ture (Comcast Applied AI Research)
- Nicola Ueffing (eBay)
- Masao Utiyama (NICT)
- Dušan Variš (Charles University, Institute of Formal and Applied Linguistics)
- David Vilar (Amazon)
- Ivan Vulić (University of Cambridge)
- Ekaterina Vylomova (University of Melbourne)
- Wei Wang (Google Research)
- Weiyue Wang (RWTH Aachen University)
- Taro Watanabe (Google)
- Philip Williams (University of Edinburgh)
- Hua Wu (Baidu)
- Joern Wuebker (Lilt, Inc.)
- Hainan Xu (Johns Hopkins University)
- Yinfei Yang (Google)
- François Yvon (LIMSI/CNRS)
- Dakun Zhang (SYSTRAN)
- Xuan Zhang (Johns Hopkins University)
ANTI-HARASSMENT POLICY
WMT follows the ACL's anti-harassment policy
Wmt Aw Integration Solutions
CONTACT
Wmt Aw Integration Services
For general questions, comments, etc. please send email to bhaddow@inf.ed.ac.uk.
For task-specific questions, please contact the relevant organisers.
Wmt Aw Integration Inc
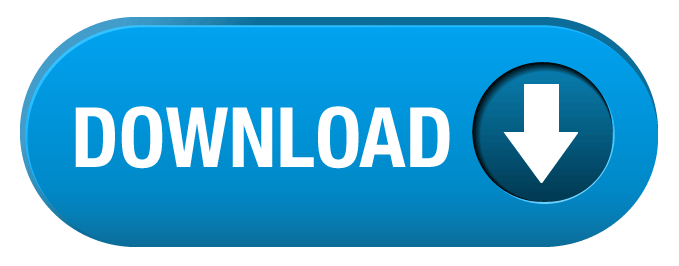